
Atmospheric correction emulation
Building an atmospheric correction emulator based on synthetic data and deep learning (2023 - 2024)
This research project, funded by the NASA Surface Biology and Geology mission, and in partnership with the NASA Ames Data Science group, aims to develop of an atmospheric correction emulator for remote sensing of multispectral and hyperspectral sensors. This innovative tool will be built using synthetic data and cutting-edge deep learning techniques. The main objective is to create a more precise and efficient method for correcting the atmospheric distortions that can affect the data retrieved by these sensors in the field of remote sensing, atmospheric correction is essential to ensure the accurate interpretation of data. As light from the Earth's surface travels through the atmosphere to a satellite sensor, it interacts with atmospheric constituents like gases, aerosols, and clouds. These interactions can distort the sensor measurements, causing them to differ from the actual properties of the Earth's surface. Hence, without proper atmospheric correction, the resultant data may lead to incorrect analyses and conclusions. Traditional atmospheric correction methods often rely on pre-existing models and in-situ measurements. However, these can be computationally intensive, may not always be precise, and require regular updates as atmospheric conditions change. By developing a deep learning-based atmospheric correction emulator, we aim to overcome these challenges. Our emulator will leverage high fidelity synthetic data from the SWIPE ecosystem, which will allow us to create an extensive, diverse, and controllable dataset. Deep learning models will then be trained on this data, learning to identify and correct the distortions caused by the atmosphere as well as provide additional corrections for other sources of uncertainty such as sunglint and the adjacency effect. The resulting system will offer a more adaptable, efficient and effective approach to atmospheric correction, increasing the precision of remote sensing data and therefore enhancing our ability to monitor and predict harmful algal blooms and water quality. This work will benefit remote sensing applications in environmental monitoring and disaster management for coastal and inland aquatic environments
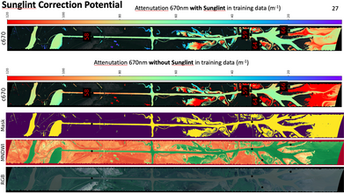
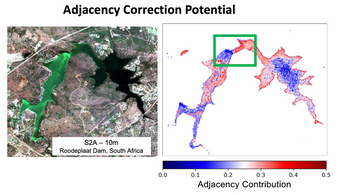