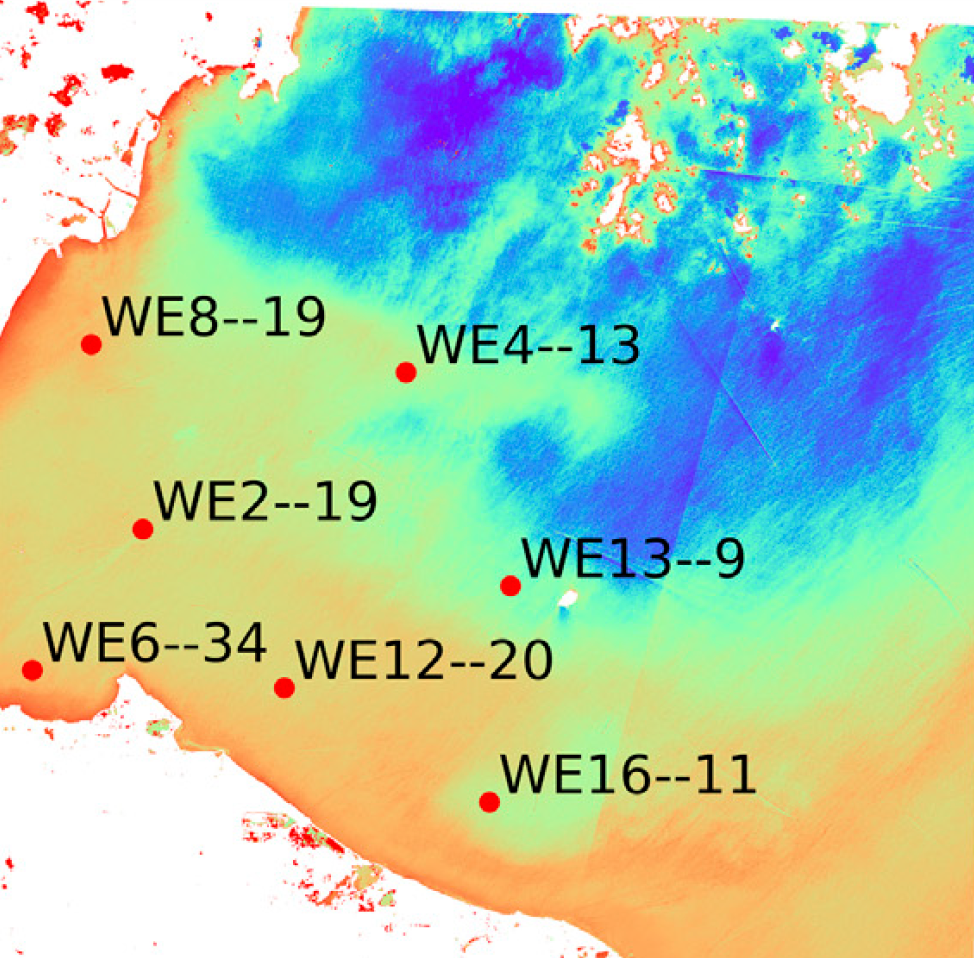
Modeling cyanobacteria with synthetic data and machine learning
Cyanobacteria modeling and estimation from multispectral sensors (2019 - 2021)
This research aims to improve how we monitor the quality of inland water (like lakes and rivers) from space using Earth Observation applications. These applications typically analyze the light reflected off the water's surface, which can provide information about what's in the water. However, developing accurate models for this purpose has been challenging due to a lack of specific data collected from the water bodies themselves. To tackle this issue, the researchers have created a new type of dataset that simulates the reflection of light from the water as seen from space (top-of-atmosphere) and from just above the water surface (bottom-of-atmosphere). This dataset is unique because it includes a wide range of potential water conditions, including different types and sizes of microscopic algae (phytoplankton) and other substances that affect light reflection. Additionally, the dataset takes into account the effect of green vegetation around the water bodies on the light reflection. Using this dataset, the researchers categorized the simulated light reflections into 13 different types, which can be related back to certain water conditions observed in real-life situations. The dataset was then used to train and test advanced machine learning models. These models can analyze the simulated light reflections and accurately estimate various water quality parameters and substances in the water across a wide range of water conditions and sensor resolutions. In essence, this research has developed new tools that can significantly improve our ability to monitor the quality of inland water on a global scale from space. These advancements can contribute to more effective and timely management of our water resources.
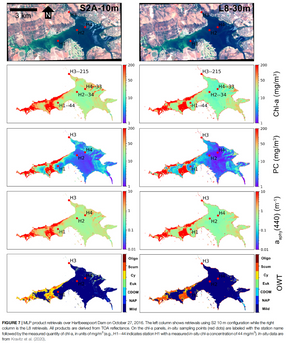
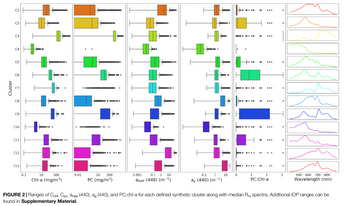
