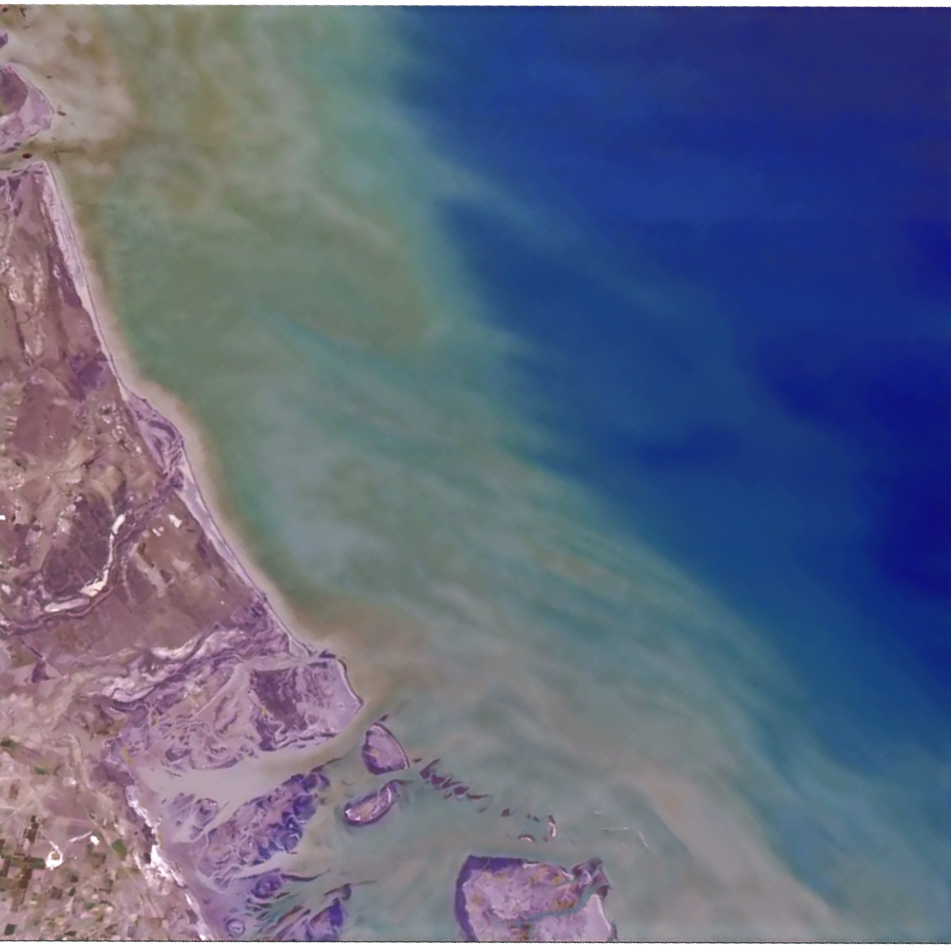
Spectral Water Inversion Processor and Emulator (SWIPE)
A one-stop-shop for advanced spectral modeling, emulation, and analysis for global aquatic ecosystem applications using remote sensing. (2021 - ongoing)
Water quality is increasingly recognized as one of society’s main challenges in the 21st century. Resulting socio-economic impacts on lives and livelihoods are particularly felt in under-developed regions, with political instability an emerging consequence of water stress. Advertent monitoring and accurate forecasting is of critical importance towards appropriate and pragmatic management of coastal and inland aquatic resources. Thus, I have been leading the development of the spectral water inversion processor and emulator (SWIPE), a holistic computational framework for advanced forward and inverse optical modeling to enable accurate and rapid Earth Observation of aquatic ecosystems. SWIPE enables production of state-of-the-art hyperspectral synthetic radiometric data paired with full optical descriptions of the aquatic and atmospheric column relevant to global ocean, coastal, and inland aquatic ecosystems. A distributed equivalent algal populations (DEAP) model employs a two-layer (coated sphere) scattering code to derive the full suite of optical properties for a variety of inorganic and organic particles from first principles. Ranges of in-water and atmospheric constituent variability are selected to represent those of natural ecosystems globally, and corresponding hyperspectral reflectances are simulated via radiative transfer modeling in the visible and near-infrared spectrum. The synthetic dataset is leveraged to train deep learning models which can emulate the complex physical interactions underlying the nonlinear processes in Earth systems and directly produce biogeophysical properties of the water column with per-pixel uncertainty. We show that deep learning models trained on SWIPE data perform as well as models trained on global experimental data. Application to a new generation of airborne and orbiting hyperspectral sensors demonstrates cross-sensor utility. Our approach is computationally efficient, sensor agnostic, generalizes to various water types found globally, and provides quantified uncertainties. The synthetic dataset, bio-optical models, and inversion algorithms are open source and expected to facilitate mission design, science discovery, scaling efforts, and maximizing retrievable information content.
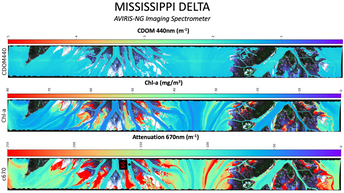
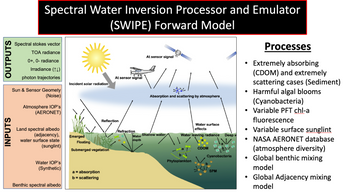

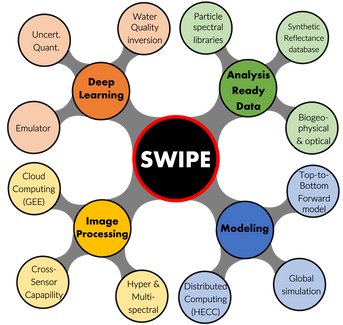